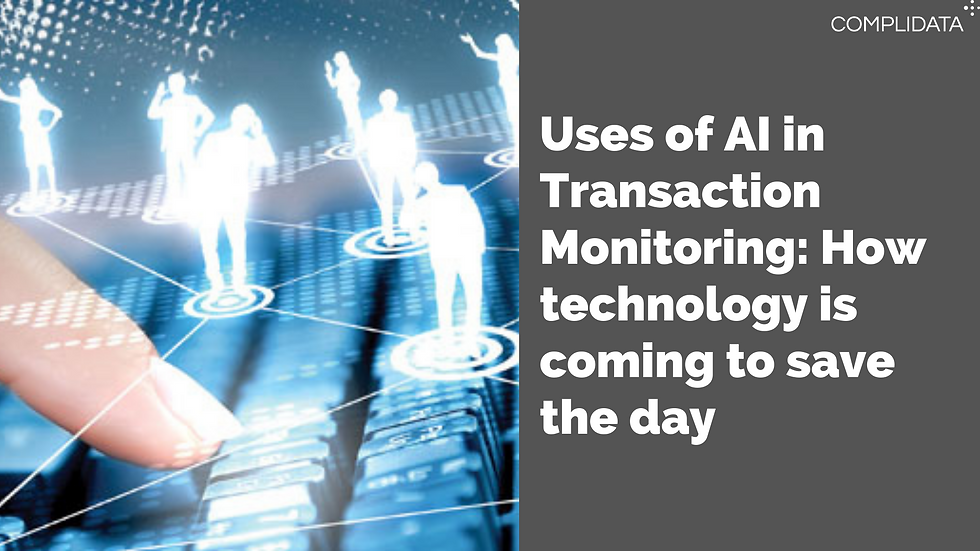
Artificial intelligence (AI) is transforming various domains and industries, and transaction monitoring is no exception. Traditional TM systems are often characterised by high false positive rates, low risk assessment accuracy, and limited explainability. In this whitepaper, Bharat Ram Ammu, Complidata’s Head of Data Science, explains how AI techniques are coming to save the day.
The whitepaper covers four key uses of AI in TM: risk scoring alerts, scenario tuning, anomaly scoring for transaction analysis, and named entity recognition. It explores in detail how Complidata's Financial Crime Risk Reduction (FCRR) is optimising and enhancing each of these four processes.
For risk scoring alerts, instead of relying solely on rule-based scenarios, FCRR leverages explainable machine learning models to generate risk scores and narratives for each alert, providing valuable insights into flagged transactions' risk factors. By using the outcome of alert investigations as a learning signal, FCRR optimizes the risk scoring process. The solution offers a remarkable 30-70% theoretically lossless reduction in false positives, leading to improved operational efficiency, cost reduction, and more focused investigations, ultimately enhancing the overall effectiveness of financial crime detection. To ensure transparency, FCRR showcases explanations and narratives with each risk score, making it accessible and user-friendly for investigators. With its advanced techniques and user-friendly approach, FCRR revolutionizes risk scoring and explainability in TM systems, providing actionable insights and facilitating efficient investigations.
Complidata offers two AI-based approaches to scenario tuning. Approach A involves scenario redesign and uses local and global ML models to provide insights and recommendations. It includes subgroup discovery and ML tree-based rules for proposing new rules to optimize existing scenarios. Approach B focuses on Below-the-Line (BTL) tuning, utilizing local and global ML models for insights and recommendations. It employs the Autotune Python tool, a multi-parameter scenario optimization tool, using a greedy search algorithm and intuitive visualizations to explore and optimize parameter combinations for enhanced risk assessment. By leveraging these approaches, Complidata empowers users to make informed decisions and improve the overall effectiveness of financial crime detection in TM systems.
Apart from risk alerts scoring and scenario tuning, FCRR also leverages an innovative approach to identify potentially anomalous transactions by combining multiple anomaly scoring techniques. This cutting-edge methodology involves the following steps:
1. Data Collection and Preparation: FCRR gathers transactional data, customer profile features, and customer summary statistics, including demographic information, historical transaction patterns, and account details.
2. Feature Engineering: Relevant features are extracted from the transactional data, incorporating transaction amounts, time intervals between transactions, and types. Customer profile features such as age, income level, and account history are also included, and customer summary statistics are generated from aggregated transactional data.
3. Class of Anomaly Scoring Models: FCRR develops a class of anomaly scoring models using diverse techniques like isolation forest, autoencoders, variation autoencoders, PCA outlier detection, and histogram-based outlier detection. These models are fitted on the input data, considering both transactional and customer profile features, to generate individual anomaly scores for each transaction.
4. Combination of Anomaly Scores: To form a unified class of anomaly scores, FCRR employs combination techniques such as ensemble methods or weighted averaging. This allows for an effective merge of individual anomaly scores, considering the strengths and weaknesses of each model.
5. Evaluation and Validation: The performance of FCRR's combined anomaly scoring approach is assessed using relevant evaluation metrics, such as precision, recall, and accuracy (F1) score. Validation is done on a representative dataset to ensure genuine anomaly detection while minimizing false positives and negatives.
6. Integration and Deployment: Once fine-tuned, the anomaly scoring models and combination techniques are integrated into the transaction monitoring system infrastructure. FCRR establishes appropriate data pipelines for real-time or batch processing and continuously monitors and refines the integrated solution.
By implementing this comprehensive methodology, FCRR effectively identifies potential anomalies and suspicious activities in transactions, providing financial institutions with a powerful tool to enhance their risk detection capabilities.
Perhaps the most head-scratching, migraine-inducing aspect of TM is named entity screening. Complidata's NER (Named Entity Recognition) tool utilizes deep learning and AI expertise to extract and resolve significant entities while detecting the country mentioned in textual data. Leveraging state-of-the-art deep learning models, it accurately identifies meaningful entities, including names, companies, and locations, enabling comprehensive understanding and analysis. The tool incorporates an algorithm that employs direct matching, fuzzy search, and a pretrained deep learning model to detect countries mentioned in the text. Through the fusion of advanced techniques and algorithms, NER ensures efficient entity extraction and precise country detection, showcasing Complidata's commitment to leveraging cutting-edge technologies for valuable insights from textual data and reinforcing their expertise in deep learning and AI applications.
Read the full whitepaper here: